Shared Molecular Neuropathology Across Major Psychiatric Disorders Parallels Polygenic Overlap
Abstract
The predisposition to neuropsychiatric disease involves a complex, polygenic, and pleiotropic genetic architecture. However, little is known about how genetic variants impart brain dysfunction or pathology. We used transcriptomic profiling as a quantitative readout of molecular brain-based phenotypes across five major psychiatric disorders—autism, schizophrenia, bipolar disorder, depression, and alcoholism—compared with matched controls. We identified patterns of shared and distinct gene-expression perturbations across these conditions. The degree of sharing of transcriptional dysregulation is related to polygenic (single-nucleotide polymorphism–based) overlap across disorders, suggesting a substantial causal genetic component. This comprehensive systems-level view of the neurobiological architecture of major neuropsychiatric illness demonstrates pathways of molecular convergence and specificity.
(Gandal et al., “Shared molecular neuropathology across major psychiatric disorders parallels polygenic overlap” Science 09 Feb 2018:Vol. 359, Issue 6376, pp. 693-697 (DOI: 10.1126/science.aad6469). Reprinted with permission from AAAS)
Despite remarkable success identifying genetic risk factors for major psychiatric disorders, it remains unknown how genetic variants interact with environmental and epigenetic risk factors in the brain to impart risk for clinically distinct disorders (1, 2). We reasoned that brain transcriptomes—a quantitative, genome-wide molecular phenotype (3)—would allow us to determine whether disease-related signatures are shared across major neuropsychiatric disorders with distinct symptoms and whether these patterns reflect genetic risk.
We first analyzed published gene-expression microarray studies of the cerebral cortex across five major neuropsychiatric disorders (3–11) using 700 cerebral cortical samples from subjects with autism (ASD) (n = 50 samples), schizophrenia (SCZ) (n = 159), bipolar disorder (BD) (n = 94), depression (MDD) (n = 87), alcoholism (AAD) (n = 17), and matched controls (n = 293) (12). These disorders are prevalent and disabling, contributing substantially to global disease burden. Inflammatory bowel disease (IBD) (n = 197) was included as a non-neural comparison.
Individual data sets underwent stringent quality control and normalization (Fig. 1) (12), including rebalancing so as to alleviate confounding between diagnosis and biological (such as age and sex) or technical (such as post mortem interval, pH, RNA integrity number, batch, and 3’ bias) covariates (figs. S1 and S2). Transcriptome summary statistics for each disorder were computed with a linear mixed-effects model so as to account for any sample overlap across studies (12). Comparison of differential gene expression (DGE) log2 fold change (log2FC) signatures revealed a significant overlap among ASD, SCZ, and BD and SCZ, BD, and MDD (all Spearman’s ρ ≥ 0.23, P < 0.05, 40,000 permutations) (Fig. 2A). The regression slopes between ASD, BD, andMDD log2-FC effect sizes compared with SCZ (5.08, 0.99, and 0.37, respectively) indicate a gradient of transcriptomic severity with ASD > SCZ ≈ BD > MDD (Fig. 2B). To ensure robustness, we compared multiple methods for batch correction, probe summarization, and feature selection, including use of integrative correlations, none of which changed the qualitative observations (fig. S3) (12). Results were also unaltered after first regressing gene-level RNA degradation metrics, suggesting that systematic sample quality issues were unlikely to drive these correlations (fig. S3). Further, the lack of (or negative) overlap between AAD and other disorders suggests that similarities are less likely due to comorbid substance abuse, poor overall general health, or general brain-related post-mortem artefacts.
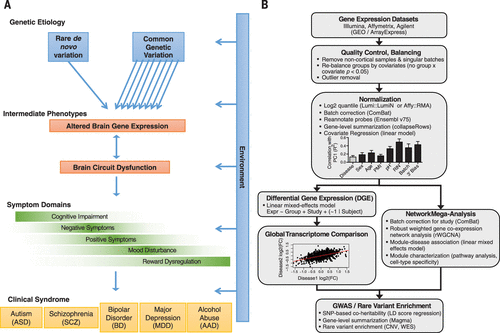
Figure 1. Experimental Rationale and Designa
a A color version of the figure, as originally published, appears in the online version of this article (focus.psychiatryonline.org).
A Model of psychiatric disease pathogenesis.
B Flowchart of the cross-disorder transcriptome analysis pipeline (12). Cortical gene expression data sets were compiled from cases of ASD (n = 50 samples), SCZ (n = 159), BD (n = 94), MDD (n = 87), AAD (n = 17), and matched non-psychiatric controls (n = 293) (table S1) (12).
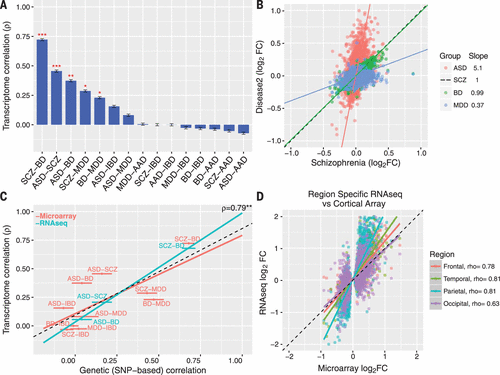
Figure 2. Cortical Gene Expression Patterns Overlapa
a A color version of the figure, as originally published, appears in the online version of this article (focus.psychiatryonline.org).
A Rank order of microarray transcriptome similarity for all disease pairs, as measured with Spearman’s correlation of differential expression log2FC values.
B Comparison of the slopes among significantly associated disease pairs indicates a gradient of transcriptomic severity, with ASD > SCZ ∼ BD > MDD.
C Overlapping gene expression patterns across diseases are correlated with shared common genetic variation, as measured with SNP coheritability (22). The y axis shows transcriptome correlations using microarray-based (discovery, red) and RNA-seq (replication, blue) data sets.
D RNA-seq across all cortical lobes in ASD replicates microarray results and demonstrates a consistent transcriptomic pattern. Spearman’s ρ is shown for comparison between microarray and region-specific RNA-seq replication data sets (all P < 10−14). Plots show mean ± SEM. *P < 0.05, **P < 0.01, ***P < 0.001.
Disease-specific DGE summary statistics (data table S1) provide human in vivo benchmarks for determining the relevance of model organisms, in vitro systems, or drug effects (13, 14). We identified a set of concordantly down-and up-regulated genes across disorders (fig. S4) as well as those with more specific effects. Complement component 4A (C4A), the top genome-wide association study (GWAS)–implicated SCZ disease gene (15), was significantly up-regulated in SCZ (log2FC = 0.23, P = 6.9 × 10−6) and in ASD [RNA sequencing (RNA-seq); log2FC = 0.91, P = 0.014] (data table S1) but not in BD, MDD, or AAD. To investigate potential confounding by psychiatric medications, we compared disease signatures with those from nonhuman primates treated with acute or chronic dosing of antipsychotic medications. Significant negative overlap (fig. S5) (12) was observed, indicating that antipsychotics are unlikely to drive, but rather may partially normalize, these transcriptomic alterations, whereas the psychotomimetic phencyclidine partially recapitulates disease signatures.
To validate that these transcriptomic relationships are generalizable, we generated independent RNA-seq data sets for replication for three out of the five disorders (fig. S6) (12). We identified 1099 genes whose DGE is replicated in ASD [odds ratio (OR) 6.4, P = 3.3 × 10−236, Fisher’ sexact test] (table S2), 890 genes for SCZ (BrainGVEX; OR 4.5, P = 7.6 × 10−155), and 112 genes for BD (BrainGVEX; OR 3.9, P = 4.6 × 10−26), which is likely due to the relatively smaller RNA-seq sample size for BD (12). We observed similarly high levels of transcriptomic overlap among ASD, SCZ, and BD and a similar gradient of transcriptomic severity (Fig. 2C and fig. S7). The SCZ and BD patterns were further replicated in the CommonMind data set, although gene-level overlap was lower (fig. S7) (12, 16). The ASD signature was qualitatively consistent across the four major cortical lobules, indicating that this pattern is not caused by regional differences (Fig. 2D).
To more specifically characterize the biological pathways involved, we performed robust weighted gene coexpression network analysis (rWGCNA) (12, 17), identifying several shared and disorder specific coexpression modules (Fig. 3). Modules were stable (fig. S8), showed greater association with disease than other biological or technical covariates (fig. S9), and were not dependent on corrections for covariates or batch effects (fig. S10). Moreover, each module was enriched for protein-protein interactions (fig. S8) and brain enhancer-RNA co-regulation (fig. S11) derived from independent data, which provides anchors for dissecting protein complexes and regulatory relationships.
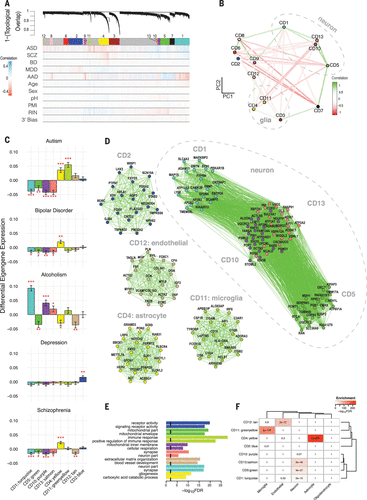
Figure 3. Network Analysis Identifies Modules of Coexpressed Genes Across Disease
A Network dendrogram from coexpression topological overlap of genes across disorders. Color bars show correlation of gene expression with disease status, biological, and technical covariates.
B Multidimensional scaling plot demonstrates relationship between modules and clustering by cell-type relationship.
C Module-level differential expression is perturbed across disease states. Plots show β values from linear mixed-effect model of module eigengene association with disease status (FDR-corrected #P < 0.1, *P < 0.05, **P < 0.01, ***P < 0.001).
D The top 20 hub genes are plotted for modules most disrupted in disease. A complete list of genes’ module membership (kME) is provided in data table S2. Edges are weighted by the strength of correlation between genes.
E and F Modules are characterized by E Gene Ontology enrichment (top two pathways shown for each module) and F cell-type specificity, on the basis of RNA-seq of purified cell populations from healthy human brain samples (25).
An astrocyte-related module (CD4 and hubs GJA1 and SOX9) was broadly up-regulated in ASD, BD, and SCZ [false discovery rate (FDR)–corrected P < 0.05] (Fig. 3C and data table S2) (12) and enriched for glial cell differentiation and fatty-acid metabolism pathways. By contrast, a module strongly enriched for microglial markers (CD11) was up-regulated specifically in ASD (twosided t test, FDR-corrected P = 4 × 10−9). Hubs include canonical microglial markers (HLA-DRA and AIF1), major components of the complement. system (C1QA and C1QB), and TYROBP, a microglial signaling adapter protein (18). Results fit with convergent evidence for microglial up-regulation in ASD and an emerging understanding that microglia play a critical role regulating synaptic function during neurodevelopment (19).
One module, CD2, was up-regulated specifically in MDD (FDR-corrected P = 0.009) (data table S2) and was enriched for G protein–coupled receptors, cytokine-cytokine interactions, and hormone activity pathways, suggesting a link between inflammation and dysregulation of the hypothalamic-pituitary (HPA) axis, which is consistent with current models of MDD pathophysiology (20). Several modules annotated as neuronal/mitochondrial were down-regulated across ASD, SCZ, and BD (CD1, CD10, and CD13) (Fig. 3C and data table S2) (12). The overlap of CD10 with a mitochondrial gene-enriched module previously associated with neuronal firing rate (21) links energetic balance, synaptic transmission, and psychiatric disease (data table S2).
The transcriptome may reflect the cause or the consequence of a disorder. To refine potential causal links, we compared single-nucleotide polymorphism (SNP)–based genetic correlations between disease pairs (22) with their corresponding transcriptome overlap. SNP coheritability was significantly correlated with transcriptome overlap across the same disease pairs (Spearman’s ρ = 0.79, 95% confidence interval 0.43 to 0.93, P = 0.0013) (Fig. 2C), suggesting that a major component of these gene-expression patterns reflects biological processes coupled to underlying genetic variation.
To determine how disease-associated variants may influence specific biological processes, we investigated whether any modules harbor genetic susceptibility for specific disorders or for relevant cognitive or behavioral traits (12). We identified significant enrichment among several of the down-regulated, neuronal coexpression modules (CD1, CD10, and CD13) for GWAS signal from SCZ and BD, as well as for educational attainment and neuroticism (FDR-corrected P < 0.05, Spearman) (Fig. 4A) (12). We also observed enrichment for the three down-regulated neuronal coexpression modules in the iPSYCH Consortium (23) ASD GWAS cohort (Fig. 4A and table S3) (12). By contrast, these modules showed no enrichment for MDD, AAD, or IBD. Further, none of the microglial- or astrocyte-specific modules showed psychiatric GWAS enrichment. Extending this analysis to disease-associated rare variants (data table S3) (2, 12), we found that the CD1 neuronal module was enriched for genes harbouring rare, nonsynonymous de novo mutations identified in ASD (OR 1.36, FDR-corrected P = 0.03, logistic regression) and SCZ cases (OR 1.82, FDR-corrected P = 0.014) but not unaffected controls (Fig. 4B). A similar CD1-enrichment was observed for genes affected by rare, recurrent copy-number variation (CNV) in ASD (OR 2.52, FDR-corrected P = 0.008) and SCZ (OR 2.46, FDR-corrected P = 0.014). These results suggest convergence of common and rare genetic variation acting to down-regulate synaptic function in ASD and SCZ.
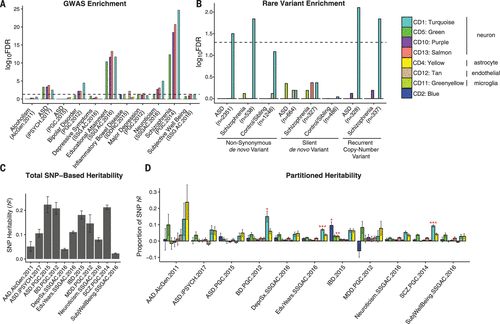
Figure 4. Down-regulated Neuronal Modules are Enriched for Common and Rare Genetic risk factorsa
a A color version of the figure, as originally published, appears in the online version of this article (focus.psychiatryonline.org).
A Significant enrichment is observed for SCZ-, ASD-, and BD-associated common variants from GWAS among neuron/synapse and mitochondrial modules (12). GWAS data sets are listed in table S3.
B The CD1 neuronal module shows significant enrichment for ASD- and SCZ-associated nonsynonymous de novo variants from whole-exome sequencing. The number of genes affected by different classes of rare variants is shown in parentheses. Significance was calculated by using logistic regression, correcting for gene length. P values are FDR-corrected.
C Total SNP-based heritability (liability scale for psychiatric diagnoses) calculated from GWAS by using LD-score regression.
D Proportion of heritability for each disorder or trait that can be attributed to individual coexpression modules. Significance (FDR-corrected *P < 0.05, **P < 0.01, ***P < 0.001) is from enrichment statistics comparing the proportion of SNP heritability within the module divided by the proportion of total SNPs represented. The CD1 module shows significant enrichment in SCZ, BD, and educational attainment.
We next used LD score regression (24) to partition GWAS heritability (Fig. 4C and data table S4) into the contribution from SNPs located within genes from each module (Fig. 4D) (12). CD1 again showed significant enrichment for SCZ (2.5-fold, FDR-corrected P = 8.9 × 10−11), BD (3.9 fold, FDR-corrected P < 0.014), and educational attainment (1.9-fold, FDR-corrected P < 0.0008; χ2 test) GWAS, accounting for ∼10% of SNP-based heritability within each data set, despite containing only 3% of the SNPs. This illustrates how gene network analysis can begin to parse complex patterns of common variants, each of small effect size, to implicate specific biological roles for common variant risk across neuropsychiatric disorders.
These data provide a quantitative, genome-wide characterization of the cortical pathology across five major neuropsychiatric disorders, providing a framework for identifying the responsible molecular signaling pathways and interpreting genetic variants implicated in neuropsychiatric disease risk. We observed a gradient of synaptic gene down-regulation, with ASD > SZ ≈ BD. BD and SCZ appear most similar in terms of synaptic dysfunction and astroglial gene up-regulation, which may represent as trocytosis, activation, or both. ASD, an early-onset disorder, shows a distinct up-regulated microglial signature, which may reflect the role for microglia in regulation of synaptic connectivity during neurodevelopment (19). MDD shows neither the synaptic nor astroglial pathology but does exhibit dysregulation of HPA-axis and hormonal signaling not observed in the other disorders.
Our data suggest that shared genetic factors underlie a substantial proportion of cross-disorder expression overlap. Given that a minority of these relationships represent expression quantitative trait loci (fig. S12), most of the genetic effects are likely acting indirectly, through a cascade of developmental and cell-cell signaling events rooted in genetic risk. Genetic variation is also not the only driver of expression variation; there is undoubtedly a contribution from environmental effects. Hidden confounders could introduce a correlation structure that matches SNP-level genetic correlations, but parsimony and hidden covariate correction suggests that this is unlikely. Diagnostic misclassification could artificially elevate shared signals, but the results are robust to disorder removal (fig. S13), and misclassification would not account for the substantial overlap we observed with ASD, which has a highly distinct phenotypic trajectory from later onset disorders. Last, we have replicated broad transcriptomic and cell type–specific patterns independently for ASD, SCZ, and BD, providing an organizing pathological framework for future investigation of the mechanisms underlying specific gene- and isoform-level transcriptomic alterations in psychiatric disease.
1 , Science 349, 1489–1494 (2015).Crossref, Google Scholar
2 : Nat. Neurosci. 19, 1397–1407 (2016).Crossref, Google Scholar
3 : Nature 474, 380–384 (2011).Crossref, Google Scholar
4 : Neurobiol. Dis. 30, 303–311 (2008).Crossref, Google Scholar
5 : PLOS Genet. 8, e1002592 (2012).Crossref, Google Scholar
6 : Mol. Psychiatry 18, 1308–1314 (2013).Crossref, Google Scholar
7 : Mol. Psychiatry 14, 1083–1094 (2009).Crossref, Google Scholar
8 , Hum. Mol. Genet. 14, 241–253 (2005).Crossref, Google Scholar
9 : Brain Res. 1239, 235–248 (2008).Crossref, Google Scholar
10 : PLOS ONE 9, e90980 (2014).Crossref, Google Scholar
11 : Mayfield, J. Neurosci. 32, 1884–1897 (2012).Crossref, Google Scholar
12 Materials and methods are available as supplementary materials.Google Scholar
13 : Trends Mol. Med. 20, 91–104 (2014).Crossref, Google Scholar
14 : Science 313, 1929–1935 (2006).Crossref, Google Scholar
15 : Nature 530, 177–183 (2016).Crossref, Google Scholar
16 : Nat. Neurosci. 19, 1442–1453 (2016).Crossref, Google Scholar
17 , BMC Bioinformatics 9, 559 (2008).Crossref, Google Scholar
18 : Cell 153, 707–720 (2013).Crossref, Google Scholar
19 , Nat. Med. 23, 1018–1027 (2017).Crossref, Google Scholar
20 , Mol. Psychiatry 20, 32–47 (2015).Crossref, Google Scholar
21 : Mol. Syst. Biol. 5, 291 (2009).Crossref, Google Scholar
22 : Nat. Genet. 45, 984–994 (2013).Crossref, Google Scholar
23 Mol. Psychiatry 23, 6–14 (2018).Crossref, Google Scholar
24 : Nat. Genet. 47, 1228–1235 (2015).Crossref, Google Scholar
25 : Neuron 89, 37–53 (2016).Crossref, Google Scholar
26 , Bioinformatics 24, 1547–1548 (2008).Crossref, Google Scholar
27 : Bioinformatics 20, 307–315 (2004).Crossref, Google Scholar
28 , Stat. Appl. Genet. Mol. Biol. 3, e3 (2004).Crossref, Google Scholar
29 , BMC Syst. Biol. 6, 63 (2012).Crossref, Google Scholar
30 , Biostatistics 8, 118–127 (2007).Crossref, Google Scholar
31 : Nat. Protoc. 4, 1184–1191 (2009).Crossref, Google Scholar
32 : BMC Bioinformatics 12, 322 (2011).Crossref, Google Scholar
33 , Bioinformatics 24, 719–720 (2008).Crossref, Google Scholar
34 : Cell 155, 1008–1021 (2013).Crossref, Google Scholar
35 : BMC Genomics 10, 327 (2009).Crossref, Google Scholar
36 : Bioinformatics 28, 2209–2210 (2012).Crossref, Google Scholar
37 , Nucleic Acids Res. 39 (suppl. 2), W307–W315 (2011).Crossref, Google Scholar
38 : Nucleic Acids Res. 38, 4218–4230 (2010).Crossref, Google Scholar
39 , J. Neurosci. 34, 1420–1431 (2014).Crossref, Google Scholar
40
41
42 : Mol. Psychiatry 18, 497–511 (2013).Crossref, Google Scholar
43 Autism Spectrum Disorders Working Group of The Psychiatric Genomics Consortium, Mol. Autism 8, 21 (2017).Crossref, Google Scholar
44 : Proc. Natl. Acad. Sci. U.S.A. 108, 7119–7124 (2011).Crossref, Google Scholar
45 : Nat. Genet. 47, 979–986 (2015).Crossref, Google Scholar
46 : Nat. Genet. 48, 624–633 (2016).Crossref, Google Scholar
47 : Nature 533, 539–542 (2016).Crossref, Google Scholar
48 : PLOS Comput. Biol. 11, e1004219 (2015).Crossref, Google Scholar
49 , Dan. Med. Bull. 44, 82–84 (1997).Google Scholar
50 : JAMA Psychiatry 71, 573–581 (2014).Crossref, Google Scholar
51 , PLOS Genet. 5, e1000529 (2009).Crossref, Google Scholar
52 , G3 (Bethesda) 1, 457–470 (2011).Crossref, Google Scholar
53 , PLOS Genet. 2, e190 (2006).Crossref, Google Scholar
54 : Bioinformatics 26, 2867–2873 (2010).Crossref, Google Scholar
55 : Gigascience 4, 7 (2015).Crossref, Google Scholar
56 : N. Engl. J. Med. 367, 1921–1929 (2012).Crossref, Google Scholar
57 : Nature 515, 209–215 (2014).Crossref, Google Scholar
58 : Nature 506, 179–184 (2014).Crossref, Google Scholar
59 : Nat. Genet. 43, 860–863 (2011).Crossref, Google Scholar
60 : Cell 154, 518–529 (2013).Crossref, Google Scholar
61 : Nature 515, 216–221 (2014).Crossref, Google Scholar
62 : Neuron 74, 285–299 (2012).Crossref, Google Scholar
63 : Nature 485, 242–245 (2012).Crossref, Google Scholar
64 : Nature 485, 246–250 (2012).Crossref, Google Scholar
65 : Lancet 380, 1674–1682 (2012).Crossref, Google Scholar
66 : Nature 485, 237–241 (2012).Crossref, Google Scholar
67 : Nat. Genet. 44, 1365–1369 (2012).Crossref, Google Scholar
68 : PLOS ONE 7, e46128 (2012).Crossref, Google Scholar
69 : Mol. Psychiatry 18, 1090–1095 (2013).Crossref, Google Scholar
70 : Neuron 87, 1215–1233 (2015).Crossref, Google Scholar
71 : Nat. Genet. 47, 291–295 (2015).Crossref, Google Scholar
72 : Nat. Neurosci. 18, 1707–1712 (2015).Crossref, Google Scholar
73 , Biostatistics 13, 204–216 (2012).Crossref, Google Scholar
74 : Proc. Natl. Acad. Sci. U.S.A. 114, 7130–7135 (2017).Crossref, Google Scholar
75 : Neurobiol. Dis. 77, 220–227 (2015).Crossref, Google Scholar
76 : Stat. Appl. Genet. Mol. Biol. 3, e29 (2004).Crossref, Google Scholar
77 : Clin. Cancer Res. 10, 2922–2927 (2004).Crossref, Google Scholar
78 : Mol. Neuropsychiatry 1, 82–93 (2015).Crossref, Google Scholar
79 : Nat. Neurosci. 18, 1168–1174 (2015).Crossref, Google Scholar
80 : Science 348, 648–660 (2015).Crossref, Google Scholar
81 : PLOS ONE 8, e56818 (2013).Crossref, Google Scholar
82 : Gut 57, 1398–1405 (2008).Crossref, Google Scholar
83 : Am. J. Hum. Genet. 92, 221–237 (2013).Crossref, Google Scholar
84 , Cell 148, 1223–1241 (2012).Crossref, Google Scholar
85 : Neuron 70, 863–885 (2011).Crossref, Google Scholar
86 : N. Engl. J. Med. 358, 667–675 (2008).Crossref, Google Scholar
87 : Am. J. Hum. Genet. 82, 477–488 (2008).Crossref, Google Scholar
88 : Am. J. Hum. Genet. 87, 618–630 (2010).Crossref, Google Scholar
89 : Nature 459, 569–573 (2009).Crossref, Google Scholar
90 : Mol. Psychiatry 21, 89–93 (2016).Crossref, Google Scholar
91 : PLOS ONE 5, e15463 (2010).Crossref, Google Scholar
92 : Nature 455, 232–236 (2008).Crossref, Google Scholar
93 : Nature 455, 237–241 (2008).Crossref, Google Scholar
94 : Am. J. Psychiatry 168, 302–316 (2011).Crossref, Google Scholar
95 : Nature 471, 499–503 (2011).Crossref, Google Scholar
96 : Hum. Mol. Genet. 18, 988–996 (2009).Crossref, Google Scholar
97 : Nat. Genet. 41, 1223–1227 (2009).Crossref, Google Scholar
98 : Am. J. Hum. Genet. 87, 229–236 (2010).Crossref, Google Scholar