Treating Refractory Mental Illness With Closed-Loop Brain Stimulation: Progress Towards a Patient-Specific Transdiagnostic Approach
Abstract
Mental disorders are a leading cause of disability, morbidity, and mortality among civilian and military populations. Most available treatments have limited efficacy, particularly in disorders where symptoms vary over relatively short time scales. Targeted modulation of neural circuits, particularly through open-loop deep brain stimulation (DBS), showed initial promise but has failed in blinded clinical trials. We propose a new approach, based on targeting neural circuits linked to functional domains that cut across diagnoses. Through that framework, which includes measurement of patients using six psychophysical tasks, we seek to develop a closed-loop DBS system that corrects dysfunctional activity in brain circuits underlying those domains. We present convergent preliminary evidence from functional neuroimaging, invasive human electrophysiology, and human brain stimulation experiments suggesting that this approach is feasible. Using the Emotional Conflict Resolution (ECR) task as an example, we show that emotion-related networks can be identified and modulated in individual patients. Invasive and non-invasive methodologies both identify a network between prefrontal cortex, cingulate cortex, insula, and amygdala. Further, stimulation in cingulate and amygdala changes patients' performance in ways that are linked to the task's emotional content. We present preliminary statistical models that predict this change and allow us to track it at a single-trial level. As these diagnostic and modeling strategies are refined and embodied in an implantable device, they offer the prospect of a new approach to psychiatric treatment and its accompanying neuroscience.
Introduction
Mental disorders are the single largest cause of disability worldwide (Whiteford et al., 2013). In the wake of recent military conflicts, they have become a major source of morbidity and mortality among warfighters and Veterans (Kessler et al., 2014; Reger et al., 2015). Disorders of concern in this population include post-traumatic stress disorder (PTSD), major depressive disorder (MDD), generalized anxiety disorder (GAD), substance use (SUD), and traumatic brain injury (TBI). Within those disorders, as with many other psychiatric diagnoses, there has been no change for decades in the overall mortality despite extensive research (Insel, 2008, 2009). Psychotropic medications adjust neurotransmitter levels globally across the brain. This fails to address the mechanisms of mental illness. If depression were a monoamine deficit, or schizophrenia a syndrome of excess dopamine, antidepressants and antipsychotics would exceed their roughly 30-40% efficacy (Lieberman et al., 2005; Warden et al., 2007; Gaynes et al., 2009; Lieberman and Stroup, 2011). The situation is worse for anxiety disorders, particularly PTSD and GAD (Thomas et al., 2010; Hoge et al., 2014). Serotonin reuptake inhibitors (SRIs), the mainstay of evidence-based pharmacology for anxiety, have modest efficacy at best (Jonas et al., 2013). Benzodiazepines (BZDs) are much stronger anxiolytics, bringing immediate short-term relief. Unfortunately, that relief can become an avoidance behavior that reinforces and worsens maladaptive responses to anxiety. BZDs also have direct addictive potential and can be rapidly fatal in overdose. Psychotherapy, particularly exposure-based therapy, has substantially greater efficacy and is often considered the true first-line treatment (Powers et al., 2010; van den Berg et al., 2015). Unfortunately, well-trained therapists are expensive and difficult for most patients to access.
More recently, a new tool has emerged: targeted electro-magnetic brain stimulation. As mental illness became understood as a dysfunction of brain circuits, investigators have sought to re-regulate those circuits (Insel, 2010; Insel and Wang, 2010). We have known for decades that targeted stereotactic lesions can be remarkably effective even in refractory cases of depression and obsessive-compulsive disorder (Kelly et al., 1973; Bingley et al., 1977; Ballantine et al., 1987; Rauch et al., 2001; Dougherty et al., 2002; Greenberg et al., 2010a; Yang et al., 2013). Stimulation may replicate the benefits of those lesions while also being reversible. Vagus nerve stimulation (VNS) and transcranial magnetic stimulation (TMS) are both approved for MDD (George et al., 2005; Aaronson et al., 2013; George et al., 2010; Johnson et al., 2013). The most promising approach, however, remains deep brain stimulation (DBS). Early open-label reports of DBS for MDD and OCD were encouraging, with high response rates even in treatment-resistant cases (Mayberg, 2009; Malone et al., 2009; Greenberg et al., 2010b). In randomized controlled trials, however, DBS for MDD failed to meet endpoints (Morishita et al., 2014; Dougherty et al., 2015). DBS for OCD is only available under a Humanitarian Device Exemption (HDE), because very few patients will ever qualify for surgery (Garnaat et al., 2014).
We attribute those failures to the neglect of a key fact: mental disorders are not static. Symptoms wax and wane across days or hours. In PTSD and anxiety disorders, they can flare and remit on the order of minutes. Standard DBS is open loop: it delivers a constant level of treatment, regardless of sleep-wake cycles, current symptom levels, or side effects. Adjustments occur only at clinical visits, weeks to months apart. This is a mis-match between the time course of the disease and the timing of clinical adjustments. One solution is closed loop stimulation, in which the device itself adjusts stimulation by inferring the patient's immediate clinical need from the brain's electrical activity (Ward and Irazoqui, 2010; Widge et al., 2014). Such approaches are clinically approved in epilepsy (Morrell, 2011), and have shown promise in Parkinson's disease (Rosin et al., 2011; Little et al., 2013). The challenge for psychiatric DBS is identifying the biomarkers – the neural signatures of mental illness and its fluctuating symptoms. Many investigators have sought these, often through neuro-imaging and electroencephalography (EEG). Despite decades of work, there is no known electrical signature of the symptoms of any mental illness. Many putative signatures do not replicate on subsequent testing (Whelan and Garavan, 2013; Widge et al., 2013; McLoughlin et al., 2014).
In response to those challenges, we describe a new approach to psychiatric DBS, funded by the Defense Advanced Research Projects Agency (DARPA) as part of the Brain Research through Advancing Innovative Neurotechnologies (BRAIN) Initiative. The essence of the TRANSFORM DBS (Transdiagnostic Restoration of Affective Networks by Systematic, Function-Oriented Real-time Modeling and Deep Brain Stimulation) project is a transdiagnostic framework. We consider psychiatric disorders as embedded in a multi-axial space of "functional domains”, similar to the National Institute of Mental Health's Research Domain Criteria (RDoC) framework (Cuthbert and Insel, 2013). We believe that these domains, being grounded in objectively measurable behavior, will have stronger and more replicable neural correlates compared to clinical psychiatric diagnoses (Widge et al., 2015). We first overview the rationale for this domain-oriented framework, identify an initial set of functional domains, and link them to disorders of national military significance. We then present a series of preliminary experiments demonstrating that these domains can be measured both non-invasively and invasively in the awake, behaving human, that the electrical and behavioral measurements are amenable to mathematical modeling, and that those models may be used to develop brain stimulation that changes psychiatrically relevant behaviors. We conclude with discussion of the next steps to turn these concepts into a clinical device. All experiments described herein were approved by the Massachusetts General Hospital Institutional Review Board and were subject to second-level review by the Army's Human Subjects Research Protection Office (HRPO).
Rationale and coverage for the transdiagnostic framework
Progress in the basic and clinical neurosciences has advanced our understanding of psychopathology. This has placed an increasing emphasis on dysfunction that cuts across domains of functioning (e.g., Negative Valence, Positive Valence, Cognitive Processes, Social Processes), as embodied in RDoC. That suggests the possibility of classifying an individual patient's mental illness based upon his/her specific patterns of dysfunction, rather than relying upon symptom clusters that show poor reliability (Regier et al., 2013). A domain-oriented approach may address the high rates of comorbidity and heterogeneity found under the checklist-based system of the Diagnostic & Statistical Manual of Mental Disorders (DSM) (American Psychiatric Association, 2013). For TRANSFORM, we have adopted six initial domains: Fear Extinction, Reward Motivation, Emotion Regulation, Decision Making/Impulsivity, Cognitive Flexibility, and Learning/Memory. These overlap with RDoC constructs, but are more specifically tailored to the clinical disorders we hope to address. Each is impaired across disorders of military interest (Table 1), each has well-established and validated metrics, and each has been linked to specific brain areas and circuits.
Functional domain | Self-report questionnaires | Task | Example disorders | Brain regions | ||||||
---|---|---|---|---|---|---|---|---|---|---|
Fear extinction | ASI* | Fear conditioning & extinction | PTSD | MDD | GAD | TBI | BPD | Pain | vmPFC, dACC, rACC, amygdala, insula, thalamus | |
ATQ* | ||||||||||
STAI* | ||||||||||
BIS/BAS* | ||||||||||
TEPS | ||||||||||
Reward motivation | BIS/BAS* | ARC | PTSD | MDD | GAD | TBI | SUD | Pain | Ventral striatum, OFC | |
PANAS* | ||||||||||
ATQ* | ||||||||||
DERS | ||||||||||
Emotion regulation | ERS | ECR | PTSD | MDD | GAD | TBI | SUD | BPD | Pain | Amygdala, rACC, dlPFC |
ASI* | ||||||||||
BIS-11 | ||||||||||
Impulsivity | ASRS | Gambling task | MDD | TBI | SUD | BPD | OFC, STN, ventral striatum | |||
BRIEF* | ||||||||||
ATQ* | ||||||||||
TRM | ||||||||||
Cognitive flexibility | BRIEF* | MSIT | PTSD | MDD | GAD | TBI | SUD | OFC, dlPFC, dACC | ||
ATQ* | ||||||||||
Learning/memory | BRIEF* | Associative learning | PTSD | MDD | GAD | TBI | SUD | dlPFC, dACC, hippocampus, thalamus, dorsal striatum |
Table 1. Functional domain-based assessment.
The domain-oriented approach resolves two related problems: diagnostic overlap and heterogeneity within single diagnoses (Fig. 1). First, patients with ostensibly different disorders may share strong common phenotypes. For example, PTSD patients frequently have deficits in Emotion Regulation, which is linked to brain connectivity between frontal regions and the amygdala (Milad et al., 2009; Rougemont-Bucking et al., 2011; Etkin et al., 2011). That dysregulation is also commonly seen in MDD (Rive et al., 2013; Heller et al., 2013), and the two disorders are often comorbid (Regier et al., 2013; Bleich et al., 1997; Campbell et al., 2007). PTSD and MDD also have a common deficit in Cognitive Flexibility: PTSD often involves perseveration on what a patient could/should have done in the moment, whereas MDD frequently has "stuck”, ruminative thinking. The strong role of the prefrontal cortex (PFC) in mental flexibility (Miller and Cohen, 2001; Buschman et al., 2012; Siegel et al., 2015; Kehagia et al., 2010) links MDD and PTSD to TBI (Fig. 1A), which also shows perseverative behavior and often involves frontal injury. Thus, a DBS intervention that targeted Cognitive Flexibility could be applicable to multiple patient groups who, from a categorical perspective do not have the "same disease”.
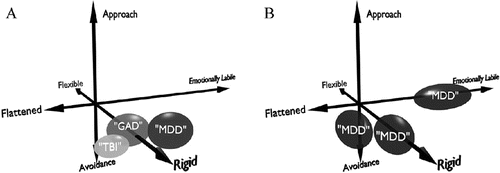
Figure 1. Schematic illustrations of transdiagnostic framework. (A), three patients with different DSM diagnoses may have the same phenotypic problem, e.g. cognitive rigidity.a
a A color version of the figure, as originally published, appears in the online version of this article (focus.psychiatryonline.org).
The other limitation of categorical diagnosis is heterogeneity. MDD is a prime example: with 9 diagnostic criteria, 5 of which must be present to confirm the diagnosis, there are 126 different clinical phenotypes, many of which have almost no symptoms in common. Individual patients with MDD may be profoundly emotionally labile (Emotion Regulation), flattened and anhedonic (Reward Motivation), or stuck in rigid, self-flagellating guilt (Cognitive Flexibility). DBS in any given brain circuit can likely only address one of those domains (Fig. 1B), suggesting that applying it to the heterogeneous construct of "depression” is predestined to find only weak clinical signals. We believe this was the key weakness in recent DBS trials in MDD, and that our domain-focused approach can overcome it (Widge et al., 2015). Put another way, patients with the same categorical diagnosis may have completely non-overlapping clinical or neurological phenotypes. The next two sections will give an example of such a situation and the ways in which the TRANSFORM approach can resolve it.
(B), three patients with MDD (notionally the "same”) may differ on the domains of cognitive flexibility, approach and avoidance to stimuli, and emotional lability.
Table 1: TRANSFORM working map between domains, assessment instruments (self-report and psychophysical), DSM-5 disorders, and implicated brain regions. Questionnaires: ASI = Anxiety Sensitivity Index, ATQ = Adult Temperament Questionnaire, STAI = State-Trait Anxiety Inventory, BIS/BAS = Behavioral Inhibition/Behavioral Activation Scale, TEPS = Temporal Experience of Pleasure Scale, PANAS = Positive and Negative Affect Scale, DERS = Difficulties in Emotion Regulation Scale, ERS = Emotion Reactivity Scale, BIS-11 = Barratt Impulsivity Scale, ASRS = Adult ADHD Self-Rating Scale, BRIEF = Brief Inventory of Executive Functioning, TRM = Trait Rumination Scale. Tasks: ARC = Aversion-Reward Conflict, ECR = Emotion Conflict Resolution, MSIT = Multiple Source Interference Task. Disorders: PTSD = Posttraumatic Stress Disorder, MDD = Major Depressive Disorder, GAD = Generalized Anxiety Disorder, TBI = Traumatic Brain Injury, SUD = Substance Use Disorder, BPD = Borderline Personality Disorder, Pain = Pain Disorders. Brain Regions: dlPFC = dorsolateral prefrontal cortex, dACC = dorsal anterior cingulate, OFC = orbitofrontal cortex, rACC = rostral anterior cingulate, STN = subthalamic nucleus, vmPFC = ventromedial prefrontal cortex.
Table 1 also illustrates that each domain maps to a suite of self-report questionnaires and a validated psychophysical task. By comparing a patient's behavior on these tasks to a cohort of healthy controls, we may create an individualized profile that identifies where a specific patient falls along an axis of adaptive versus maladaptive functioning within each domain. That multimodal assessment includes diagnostic interviews, questionnaires, behavioral data, and functional neuroimaging. The process begins with a clinical diagnostic interview (e.g., the Structured Clinical Interview for DSM), which is still necessary to validate our emerging results against established clinical practice. The results of that interview and a battery of self-report questionnaires then guide our analysis of imaging and psychophysical data. Fig. 2 presents transdiagnostic assessment results for two patients who completed our protocol, compared against a normative database of seventeen healthy controls who completed the same fMRI protocol and who had no psychiatric or medical disorders by self-report. (See SI Methods for more detail on fMRI acquisition and analysis.) Both patients had primary diagnoses of bipolar disorder and were in a major depressive episode. Patient 1 had an additional diagnosis of GAD, and Patient 2 had an additional diagnosis of PTSD. Both showed deviations from normal functioning on measures of emotion regulation and cognitive flexibility. Patient 2, however, had higher negative affect scores, whereas Patient 1 was within population norms. The reverse was true for measures of positive affect and reward motivation. These differences highlight the heterogeneity and functional overlap within a single syndrome such as clinical depression.
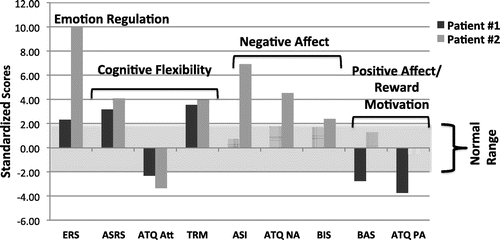
Figure 2. Transdiagnostic psychological assessment for two patients diagnosed with bipolar depression. Shaded bar represents normal range, within 2 standard deviations from standardized means of healthy control cohort. Abbreviations as per Table 1.a
a A color version of the figure, as originally published, appears in the online version of this article (focus.psychiatryonline.org).
Phenotyping psychiatric patients with transdiagnostic tasks and imaging
Behavioral phenotypes may help classify patients, but do not show where or how to intervene. Intervention requires identification of the brain network(s) that drive dysfunction in each domain, which can then be targeted with brain stimulation. We plan to localize those networks spatially with functional magnetic resonance imaging (fMRI) and temporally with magnetoencephalography/electroencephalography (MEG/EEG). Our example patients both showed difficulty with emotion regulation, as measured by the Emotional Reactivity Scale (ERS). A well-defined task for measuring this is the Emotional Conflict Resolution (ECR) task (Etkin et al., 2006, 2010, 2011). The ECR was developed as an affective analog of the Stroop task. Subjects are required to report on the emotion expressed by photographed faces (fearful or happy facial expressions) while ignoring a written word appearing as type across the photograph ("Happy” or "Fear”; Fig. 3). The written words either match the expression of the face (congruent, or "C” trials, e.g. a fearful face with the word "Fear” written across it) or do not (incongruent, or "I” trials, e.g. a fearful face with the word "Happy” written across it). Performances on trials in this task are history dependent, such that the neural and behavioral response to either a congruent or incongruent image is affected by the image presented immediately prior. These differences represent the recognition of emotion conflict when a congruent trial is followed by an unexpected incongruent trial, or the regulation of emotion conflict when incongruent images are presented one after another. Different neural circuits are recruited during emotion detection based on the presence or absence of emotional conflict in the prior trial (Heller et al., 2013). The task therefore models patients' ability to regulate context-irrelevant affective information in the service of meeting ongoing goal-directed demands.
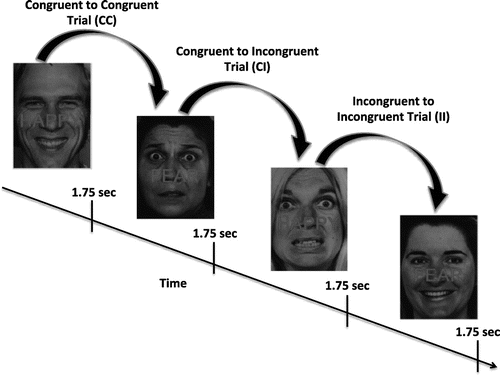
Figure 3. Trial and trial-switch types in the Emotion Conflict Resolution (ECR) task (Etkin et al., 2006). Subjects judge the facial emotion and attempt to ignore the word during Congruent (C) trials and incongruent (I) trials. Switching from congruent to incongruent (Cl) invokes top-down networks for conflict recognition, whereas sequential incongruent trials (II) depend on networks of emotion and conflict regulation.a
a A color version of the figure, as originally published, appears in the online version of this article (focus.psychiatryonline.org).
The ECR task has been validated in multiple fMRl studies. Healthy individuals show greater reaction times in response to incongruent relative to congruent trials, indicating an expected interference effect of emotion conflict. In addition, healthy individuals exhibit slower reaction times on conflict recognition (CI) trials in which they first encounter the "surprise” of incongruence, compared to conflict regulation (II) trials in which this initial response to conflict is overcome in order to continue meeting task demands. Slower reaction times during conflict recognition are associated with additional top-down effort, reflected in greater recruitment of the amygdala, dorsomedial prefrontal cortex (dmPFC), and dorsolateral prefrontal cortex (dlPFC) during conflict recognition (CI trials). By contrast, conflict regulation (II) trials are associated with greater regulatory recruitment of the rostral anterior cingulate cortex (rACC) and increased negative coupling between rACC and amygdala, with increases in rACC activity predicting decreases in amygdala activity, indicating successful regulation of emotion conflict.
As an example of a domain-based imaging diagnostic, we used these prior results to develop an ECR-based profiling of emotion regulation in two psychiatric patients. Below, we present a "first-pass” look at singlepatient data from these patients as a proof of concept of this approach. Optimizing this for a statistically rigorous comparison of single-patient activations against a cohort of healthy controls is a focus of ongoing work.
Fig. 4 shows first-level conflict recognition (CI) > conflict regulation (II) contrasts, computed from a general linear model (GLM; see SI methods). In this preliminary inspection, both patients deviated from activation patterns found in healthy controls, but did so in unique ways (Fig. 4a). For example, during conflict recognition trials as compared to conflict regulation, healthy controls show robust bilateral recruitment of dmPFC, dlPFC, vlPFC and anterior insula. The majority of healthy controls to date (>60%) have also shown increased amygdala activation in the CI > II contrast, suggesting more effective down-regulation of the amygdala during conflict regulation trials relative to conflict recognition (Fig. 4b). Neither Patient 1 nor Patient 2 show this differential CI > II amygdala activation, suggesting a failure to adaptively regulate amygdala response during repeated incongruent trials. Patient 1 shows less pronounced vlPFC, dmPFC, and dACC recruitment, and more pronounced dlPFC recruitment during conflict recognition trials relative to conflict regulation trials. Patient 2 shows increased dmPFC and insula recruitment during conflict recognition trials relative to conflict regulation trials, similar to healthy controls. That patient also had weaker dlPFC and increased rACC recruitment during conflict regulation relative to conflict recognition, opposite to patterns seen both in our cohort of healthy controls and in the literature.
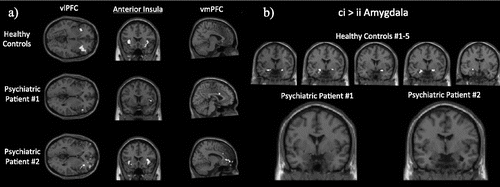
Figure 4. CI > II contrast results. (A) generalized linear model (GLM) results for healthy controls and individual GLM fixed effects for two psychiatric patients. (B) Individual GLM fixed effects in amygdala activation for five representative healthy controls and two psychiatric patients. Highlighted voxels indicate p < 0.05 uncorrected.a
a A color version of the figure, as originally published, appears in the online version of this article (focus.psychiatryonline.org).
We next used psychophysiological interaction (PPI) analyses to identify network changes that may drive patients' behavior. In the recognition vs. regulation (CI > II) contrast, we found a broad pattern of weaker connections among rACC, vmPFC, dlPFC, vlPFC, and dmPFC in both psychiatric patients relative to those found in healthy controls. Functional connectivity patterns also varied between the two patients. For example, both patients show weaker vmPFC-amygdala connectivity than connectivity in healthy controls, but Patient 2 shows substantially weaker functional connectivity than Patient 1 (Fig. 5A). Patient 2, by contrast, shows stronger dlPFC-amygdala functional connectivity than that seen in healthy controls, while Patient 1 has an opposite pattern (Fig. 5B). This illustrates our point that patients with the same categorical diagnosis may have diametrically opposite brain impairments, necessitating a new approach to classifying their specific circuit deficits.
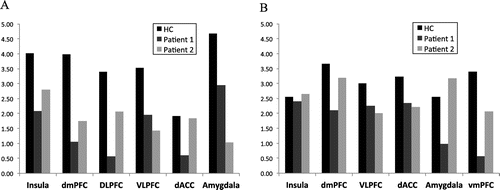
Figure 5. Functional connectivity from fMRI imaging. Results show psychophysiological interaction z-scores for the CI > II contrast in brain regions of interest. (A) vmPFC seed region and (B) dlPFC seed region.a
a A color version of the figure, as originally published, appears in the online version of this article (focus.psychiatryonline.org).
These results suggest the feasibility of transdiagnostic patient classification, but are not sufficient to create a closed-loop system. The high spatial resolution of MRI shows where in the brain a behaviorally-relevant neural dysfunction may reside, but the relatively poor temporal resolution does not reveal the what or when of this dysfunction. That requires a deeper understanding ofthe temporal dynamics within and between distributed brain regions. Under TRANSFORM, we are collecting that information through invasive human recordings and MEG/EEG in non-surgical patients. The following section will illustrate how those time-resolved techniques might link with fMRI to yield a deeper picture of emotion regulation.
Linking “where” to “what” via human invasive electrophysiology
Closed-loop brain stimulation requires an understanding of how behavior and/or psychological states are encoded moment-to-moment in brain activity, which cannot be captured in fMRI's single snapshots. Electrophysiology can show us "what” neural ensembles are doing at the locations "where” the fMRI results have detected emotional conflict processing. These physiological dynamics need to be understood to modulate the network and identify therapeutically relevant states. Here, we illustrate those dynamics using human LFPs recorded with sub-acutely implanted electrodes during clinical evaluation of patients with intractable epilepsy (Lachaux et al., 2000). Such data have been used to study the mechanisms of a wide variety of cognitive capabilities (Lachaux et al., 2000; Dykstra et al., 2011; Chan et al., 2014). TRANSFORM uses those recordings and our transdiagnostic task battery to link imaging to physiology.
Electrode placement, although driven purely by clinical criteria, often targets the same areas implicated by our trans-diagnostic battery. This allows us to understand LFP dynamics in regions indicated by fMRI. In one prototypical example, a 55-year-old woman whose epilepsy was thought to be related to a left insular dysplasia performed the ECR task while awaiting seizure localization (Fig. 6). Electrode locations were reconstructed based on post-operative CT scans co-registered with preoperative MR1 (Dykstra et al., 2012), verifying that we had accessed areas showing ECR-related fMRI activation in our healthy control cohort (Fig. 6A). To examine event-related potentials (ERPs), intracranial LFPs were downsampled from 30 kHz to 1 kHz. Line noise (60, 120, and 180 Hz) was removed by channel-wise band-pass filtering and subtraction. Trials with excessive artifact were rejected by visual inspection. The average ERP across trials and its standard error were then computed channel-wise.
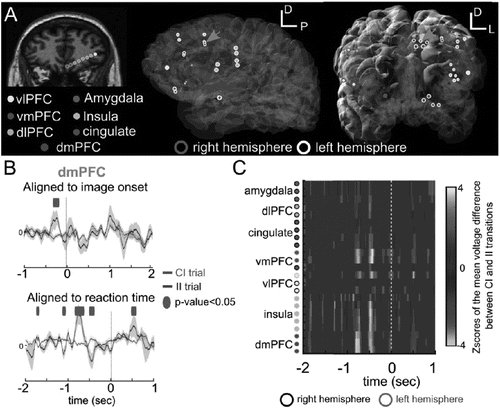
Figure 6. Invasive human electrophysiology during the ECR task. (A), 3-dimensional reconstructions of clinical depth electrodes from fusion of preoperative MRI and postoperative CT. For clarity, the sagittal and coronal views show only those electrodes that correspond to regions identified in fMRI analyses. Colors indicate different brain regions as per the legend. D-dorsal, L-lateral, P-posterior. (B), event-related potentials (ERPs) in the dmPFC, time-locked to stimulus onset (upper) or response (lower). Electrode is indicated by an arrowhead in (A). Red dots indicate significant differences between II and CI trials, false discovery rate controlled per channel. (C), Z-score of (CI - II) ERP difference across brain regions, time-locked to response (white dashed line), masked to show only |Z| > 1.5. The period between 1000 and 500 ms before the response shows strong ERP differences across channels, particularly in insula and medial PFC bilaterally.a
a A color version of the figure, as originally published, appears in the online version of this article (focus.psychiatryonline.org).
Regions implicated by fMRI also showed significant ERP modulation in response to the ECR stimuli. For example, in dmPFC, a window of approximately 1000 to 500 ms before the response had significant conflict-regulation (II > CI) differences (Fig. 6B; one-way ANOVA; n = 7 II trials; n = 20 CI trials, false discovery rate corrected for multiple timepoints). This is opposite in sign to the fMRI finding, suggesting that the BOLD signal may represent metabolic activity from inhibitory processes during conflict recognition (CI sequences). We did not observe similar large windows of ERP difference in a stimulus-locked ERP analysis (Fig. 6B). To examine this effect across regions, we computed the (CI - II) difference for ERPs at multiple electrodes in fMRI-identified regions (Fig. 6C). To correct for multiple comparisons across time and space, we computed the null distribution of this multichannel difference by randomly permuting the trial labels 1000 times and converting the differences into a Z-score (Fig. 6C). We found strong responses (Z > 1.96, p < 0.05) in the insula, amygdala, cingulate, vlPFC, vmPFC, dlPFC, and dmPFC. We therefore found a correspondence between neurophysiological activity, in the form of ERPs, and our fMRI findings (Fig. 4). This is somewhat surprising, as ERP and fMRI activations do not necessarily arise from the same mechanism, although they may have some common generators (Vitacco et al., 2002; Fan et al., 2007; Herzmann et al., 2012). These results nevertheless suggest that "where” the neural activity occurs can be mapped with the fMRI and then resolved at the neural population level with LFP.
Changes in LFP network dynamics during ECR (Fig. 7) also localize to the same structures implicated by PPI analyses (Fig. 5). We examined LFP network connectivity by measuring the trial-averaged coherence within frequency bands and between all pairs of channels (Oostenveld et al., 2010). Focusing on the identified significant window of 1000 to 500 ms before the reaction time, we computed the (CI - II) difference, estimated a null distribution of that difference by 1000 random permutations of the trial labels, and converted the observed coherence to a Z-score against that null distribution. In this patient, we observed band-specific, trial-driven differences in connectivity (Fig. 7). II trials had greater coherence at 8-15 Hz (alpha band) between cingulate, amygdala, insula and dlPFC (Fig. 7B). CI trials also had increased coherence between frontal structures and insula, but in the high-gamma 65-100 Hz band (Fig. 7E). We thus identify the same structures called out in preliminary fMRI analyses, but with an interesting additional possibility: different frequency bands may serve top-down vs. bottom-up communication, or may reflect rule performance (II) as opposed to rule switching (CI). Other investigators have found rule-switching effects in PFC in the alpha-band, although linked to local oscillatory power, not coherence (Buschman et al., 2012).

Figure 7. Coherence between channel pairs is frequency-specific during the ECR task. Coherence for each pair is represented as a permutation-derived Z-score of the mean coherence difference between CI and II trials from 1000 to 500 ms before the button-press response. All values are masked at |Z| > 1.5 to emphasize differences. (A) 4-8 Hz, (B) 8-15 Hz, (C) 1530 Hz, (D) 30-55 Hz, (E) 65-100 Hz. II trials showed higher fronto-insular coherence in the alpha frequency band (B). In contrast, CI trials had higher coherence values in the same regions but in the high gamma band. The dots along the x and y axes indicate brain regions based on color and whether the channels were in the left or right hemisphere (see legend).a
a A color version of the figure, as originally published, appears in the online version of this article (focus.psychiatryonline.org).
Overall, these findings suggest that network impairments detected with non-invasive assessment correlate to areas with significant electrophysiologic modulation in response to the same task. It should therefore be possible to use the behavioral and imaging pipelines to design a patient-specific set of implant targets to maximize signal detection for closed-loop applications.
Modeling the effects of brain stimulation interventions
The MRI and LFP data indicate that a single task can engage multiple brain regions in complex ways and may evoke behavior that depends on past trials. Closed-loop control requires lower-dimensional mathematical models of how brain and behavior respond to external drivers such as brain stimulation. In this section, we develop a model to predict the network effects of DBS-like stimulation. We will then link that to behavioral change in the next section.
Stimulation effects can be modeled in causal dynamical systems frameworks (Franaszczuk and Bergey, 1998; Kramer et al., 2010; Martin et al., 2014), from linear multivariate autoregressive (MVAR) models to non-linear dynamic causal models (Murta et al., 2012; Basu et al., 2015). Such models may capture differences in network "wiring” under different trial conditions, including the presence/absence of brain stimulation. Here, we present an example using a general linear multivariate (gMVR) network model. A gMVR is of the form:

Where, Y is the series of multivariate measurements, X is the matrix of predictor variables, β is the matrix of parameters to be estimated, and E is multivariate white noise. Similar models form the basis of Granger causality analysis (Wilke et al., 2008; Ding et al., 2011; Barnett and Seth, 2014). A standard MVAR model, however, uses a fixed and low-order structure (generally 5-10 lags) for both the local activity within a brain structure (self history) and the propagation of activity between structures (cross history). This is not biophysically correct, as both axonal conduction and synaptic transmission can introduce tens of milliseconds of delay between areas. Hence, we adapted the framework to have a short-term self history and longer, independently chosen time lags for cross history.
We fit this gMVR model using LFP data from the right vmPFC, dACC, and amygdala in the same patient as Fig. 6, incorporating a simple forcing function representing stimulation at each node (SI Methods). The model predicts different effects depending on both the stimulated node and the trial type (Fig. 8A). Perturbing vmPFC or dACC during CI trials is overall less effective than stimulating during II trials. dACC stimulation (increase in gamma power) tends to inhibit vmPFC regardless of the trial type, whereas the effects of amygdala or vmPFC stimulation can be positive, negative, or neutral depending on recent trial history.
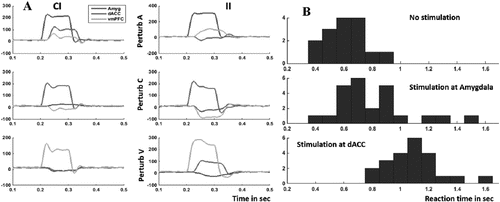
Figure 8. General multivariate regression models for simulating band limited LFP power. (A) Simulation of the effects of perturbation (in the form of an additive term to one of the nodes) at amygdala (blue, A), dACC (red, C) and vmPFC (yellow, V) for CI and II trials. (B) reaction times of CI trials with no stimulation and with 160 Hz/6 mA stimulation at A and at C.a
a A color version of the figure, as originally published, appears in the online version of this article (focus.psychiatryonline.org).
This basic model shows evidence of being able to predict stimulation outcomes. It suggests that the strongest effect would be found from dACC stimulation, which should inhibit both vmPFC and amygdala, leaving the patient unable to effectively process stimuli or regulate emotion. On a second experimental day with the same patient, we delivered high-frequency stimulation to dACC or amygdala on 50% of ECR trials just after the stimulus appeared (SI Methods). dACC stimulation produced a reaction time increase, consistent with the network disruption predicted by the gMVR model (Fig. 8B).
Based on these encouraging results with a relatively simple model, we hope to achieve more robust and wide-ranging predictions by expanding it to more detailed biophysics. Another future direction will extend the behavioral result, seeking a relationship between specific neural changes (spectral/time domain) and behavior. By reversing that relationship, we might predict what needs to be changed in the neural domain (via stimulation) to steer the network's behavioral output towards a therapeutic target.
State-space behavior modeling to capture effects of brain stimulation
Brain stimulation in this emotion-regulation network does more than simply increase reaction time (RT). Fig. 9 shows the reaction time effect of Fig. 8B at the single trial level. Both RT and fraction of trials correct change in response to both stimulation and the task factors. Stimulation does not differentially affect CI and II trials (Fig. 9B, C), but does cause trials with a Fear (F) valence to have lower performance and higher reaction times under stimulation. This effect may be more pronounced for dACC stimulation. There also appears to be a general trend superimposed on each block's effect. For instance, RT in the fourth block (second amygdala stimulation experiment) rises sharply in the beginning of the block, then drops.
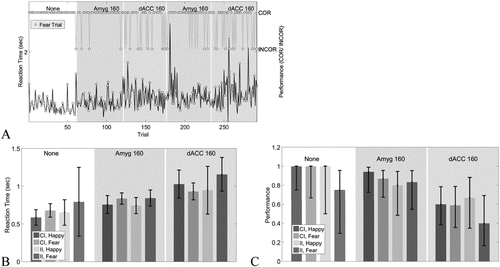
Figure 9. Behavioral summary of ECR under brain stimulation. (A) Reaction time and performance (fraction correct) at the single-trial level with multiple sites of stimulation. (B) Reaction time per stimulation block. (C) Performance per stimulation block. Broadly, stimulation appears to affect trials with a fearful face more than trials with a happy face, regardless of site. CI trials do not appear to differ from II trials in reaction time or performance unless a fearful face is present.a
a A color version of the figure, as originally published, appears in the online version of this article (focus.psychiatryonline.org).
These time-varying behavioral effects and interactions can be summarized in a low-dimensional representation. State-space models consider multiple outputs (e.g., RT and performance) to be common reflections of an underlying hidden state. The state-space approach has successfully modeled low-dimensional dynamic features of both neural and behavioral signals for a variety of cognitive tasks, including learning and Stroop-like tasks (Smith and Brown, 2003; Smith et al., 2004; Srinivasan et al., 2006; Prerau et al., 2008; Yousefi et al., 2015). Here, we define the state as the patient's response to F trials (SI Methods). The higher the state, the more the subject is affected and distracted by fear. When we estimate the maximum-likelihood value of the state process through an expectation-maximization (E-M) algorithm as per (Prerau et al., 2008; Yousefi et al., 2015), we obtain a single variable summarizing the effects of brain stimulation on decisions, reaction time, and overall fear responsiveness (Fig. 10). With stimulation in either dACC or amygdala, the state increases, tracking an increase in RT and reduction in performance. Interestingly, the result is step-like: any brain stimulation moves the system to a new state that persists even on unstimulated trials. The state-space analysis also confirms the gMVR results of the preceding section: the largest effects arise from stimulation in dACC.
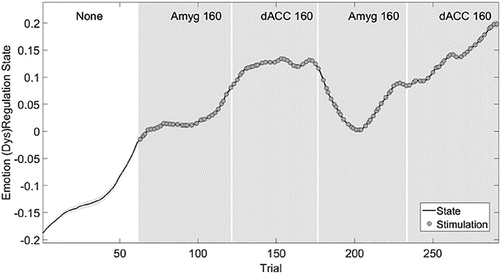
Figure 10. Cognitive state prediction in response to brain stimulation. The state variable, which reflects emotional lability to fear, increases in response to brain stimulation. Amygdala generally causes smaller changes than dACC. Both stimulation types appear to cause a step/plateau response in the state, despite being delivered on only half of the available trials.a
a A color version of the figure, as originally published, appears in the online version of this article (focus.psychiatryonline.org).
More importantly, this approach is not limited to ECR or Gaussian-distributed variables. In Yousefi et al. (2015), we describe an extension to gamma-distributed data. The behavioral output of all six domain-linked tasks can be tracked through this latent-variable approach once a theoretically valid model is formulated. This should make neural decoding for closed-loop control substantially simpler: the state variable substitutes for the velocity/position seen in a traditional brain-computer analysis, with similarly smooth temporal dynamics.
Discussion and conclusion
In our vision of next-generation psychiatric DBS, a patient will proceed through a clinical work-up based on the transdiagnostic framework (Fig. 11), and their brain stimulator will be targeted and programmed based on that framework. We have shown preliminary evidence that this pipeline is possible. This begins with neuro-imaging and behavioral assessment. In our framework, two patients who had the same clinical diagnosis (depressive episode) were more clearly separable in terms of their specific impairments. In a different patient, pathology might not take the form of visibly impaired behavior. Instead, it may appear as "compensatory activation” in electro-magnetic imaging, where patients use a wider set of brain areas (and thus detract from other ongoing processing) to maintain behavioral performance.
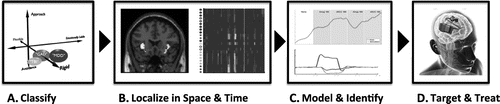
Figure 11 TRANSFORM DBS pipeline. An individual patient's dysfunction is classified in trans-diagnostic space (A). The associated brain activations are localized (B), and their relation to behavior identified through cognitive state modeling (C). Those models also predict the brain and behavioral response to applied perturbations such as electrical stimulation. From this assessment, we hope to design a patient-specific closed-loop therapy to be delivered through an integrated, multi-point closed-loop system (D).a
a A color version of the figure, as originally published, appears in the online version of this article (focus.psychiatryonline.org).
To link those activations to a patient-customized closed-loop controller, we must move from the spatial (anatomic) to the temporal domain. A key aspect of TRANSFORM is behavioral assessment while measuring brain activation with multiple modalities, both invasive and non-invasive. We showed an example from one of our first recordings in the epilepsy monitoring unit, where the same brain areas that showed high BOLD signal were recorded in a human patient with electrodes that had been implanted for seizure location. ERP and coherence analyses found changes in the same areas identified with fMRI, but resolved them to a particular time window relative to the response and to specific frequency bands.
Controlling those electrical and behavioral signatures requires a model of the "transfer function” between the brain's inputs (task cues and stimulation) and its outputs (symptoms and behavior). We presented two complementary routes toward this understanding. First, we showed that through a gMVR method, we may identify differences in brain network structure that correlate to task performance. Second, we presented initial progress toward a low-dimensional state-space approach to modeling the behavioral effects of stimulation. Expressing control in this state-space framework may simplify the process of controller development, since it allows us to simultaneously account for the effect of multiple task variables on multiple behavior outputs. In particular, we showed that amygdala and cingulate stimulation both affect behavior, but in different ways that are captured by the state-space model. While this example showed a behavioral impairment (increased reaction time and decreased number of correct trials), the same framework could capture behavioral improvement caused by stimulation at another site.
The final challenge is bringing these components together into a usable clinical device. That requires not only neural decoding and control algorithms based on these findings, but hardware that can embody those algorithms and pass regulatory approval. Given the effort and expense of qualifying implantable hardware for human use, it would be very desirable if that platform were sufficiently flexible to enable a variety of future studies. Unfortunately, no current clinical DBS system can access more than two sites at once, and stimulation linked to recordings remains an investigational feature (Afshar et al., 2013; Stypulkowski et al., 2014). To bring our work to the clinic, we are collaborating with a multidisciplinary engineering team to design a next-generation DBS system. As described more fully elsewhere (Bjune et al., 2015; Wheeler et al., 2015), this platform will be capable of stimulating four different brain sites while recording from all of those plus an optional fifth site. It includes a reconfigurable processing architecture suitable for implementing these algorithms. Power and battery will continue to be driving concerns, but careful choice of neural features should mitigate this.
In summary, although DBS for mental illness has faced hurdles, we believe the future is bright. There are major opportunities to re-consider the design and targeting of those systems now that the open-loop strategy has been proven inadequate. The TRANSFORM framework offers a way forward, based on an emerging understanding that psychiatric impairment often does not respect diagnostic boundaries. Our work in coming years will test the preliminary findings above and refine them for eventual clinical use.
Appendix A. Supplementary data
Supplementary data to this article can be found online at https://doi.org/http://dx.doi.org/10.1016/j.expneurol.2016.07.021.
2013. Vagus nerve stimulation therapy randomized to different amounts of electrical charge for treatment-resistant depression: acute and chronic effects. Brain Stimul. 6, 631–640. https://doi.org/http://dx.doi.org/10.10167j.brs.2012.09.013.Crossref, Google Scholar
,2013. A translational platform for prototyping closed-loop neuromodulation systems. Front. Neural Circuits 6,117. https://doi.org/http://dx.doi.org/10.3389/fncir.2012.00117.Crossref, Google Scholar
,1987. Treatment of psychiatric illness by stereotactic cingulotomy. Biol. Psychiatry 22, 807–819.Crossref, Google Scholar
,2014. The MVGC multivariate Granger causality toolbox: a new approach to Granger-causal inference. J. Neurosci. Methods 223, 50–68. https://doi.org/http://dx.doi. org/10.1016/j.jneumeth.2013.10.018.Crossref, Google Scholar
,2015.A study of the dynamics of seizure propagation across micro domains in the vicinity of the seizure onset zone. J. Neural Eng. 12, 046016. https://doi.org/http://dx.doi.org/10.1088/1741-2560/12/ 4/046016.Crossref, Google Scholar
,1977. Long-Term Results of Stereotactic Capsulotomy in Chronic Obsessive Compulsive Neurosis. Neurosurg. Treat. Psychiatry Pain Epilepsy. University Park Press, pp. 287–289.Google Scholar
,2015. Package Architecture and Component Design for an Implanted Neural Stimulator with Closed Loop Control. Eng. Med. Biol. Soc. EMBC. 37th Annu. Int. Conf. IEEE 2015. IEEE, pp. 7825–7830.Google Scholar
,1997. Post-traumatic stress disorder and depression. An analysis of comorbidity. Br. J. Psychiatry 170, 479–482.Crossref, Google Scholar
,2012. Synchronous oscillatory neural ensembles for rules in the prefrontal cortex. Neuron 76, 838–846. https://doi.org/http://dx.doi.org/10.1016/j.neuron.2012.09.029.Crossref, Google Scholar
,2007. Prevalence of depression-PTSD comorbidity: implications for clinical practice guideline and primary care-based interventions.]. Gen. Intern. Med. 22, 711–718. https://doi.org/http://dx.doi. org/10.1007/s11606-006-0101 -4.Crossref, Google Scholar
,2014. Speech-specific tuning of neurons in human superior temporal gyrus. Cereb. Cortex 24, 2679–2693. https://doi.org/http://dx.doi.org/10.1093/cercor/bht127.Crossref, Google Scholar
,2013. Toward the future of psychiatric diagnosis: the seven pillars of RDoC. BMC Med. 11, 126. https://doi.org/http://dx.doi.org/10.1186/1741-7015-11-126.Crossref, Google Scholar
,2011. Analyzing Coherent Brain Networks with Granger Causality. Annu. Int. Conf. IEEE Eng. Med. Biol. Soc. EMBC 2011. IEEE, Boston, MA, pp. 5916-5918. https://doi.org/http://dx.doi.org/10.1109/IEMBS.2011.6091463.Google Scholar
,2002. Prospective long-term follow-up of 44 patients who received cingulotomy for treatment-refractory obsessive-compulsive disorder. Am. J. Psychiatry 159, 269–275. https://doi.org/http://dx.doi.org/10.1176/appi.ajp.159.2.269.Crossref, Google Scholar
,2015. A randomized sham-controlled trial of deep brain stimulation of the ventral capsule/ventral striatum for chronic treatment-resistant depression. Biol. Psychiatry 78, 240–248. https://doi.org/http://dx.doi.org/10.1016/j.biopsych.2014.11.023.Crossref, Google Scholar
,2011. Widespread brain areas engaged during a classical auditory streaming task revealed by intracranial EEG. Front. Hum. Neurosci. 5, 1–14. https://doi.org/http://dx.doi.org/10.3389/fnhum.2011.00074.Crossref, Google Scholar
,2012. Individualized localization and cortical surface-based registration of intracranial electrodes. NeuroImage 59, 3563–3570. https://doi.org/http://dx.doi.org/10.1016/j.neuroimage.2011.11.046.Crossref, Google Scholar
,2006. Resolving emotional conflict: a role for the rostral anterior cingulate cortex in modulating activity in the amygdala. Neuron 51, 871–882. https://doi.org/http://dx.doi.org/10.1016/j.neuron.2006.07.029.Crossref, Google Scholar
,2010. Failure of anterior cingulate activation and connectivity with the amygdala during implicit regulation of emotional processing in generalized anxiety disorder. Am. J. Psychiatry 167, 545–554.Crossref, Google Scholar
,2011. Emotional processing in anterior cingulate and medial prefrontal cortex. Trends Cogn. Sci. 15, 85–93. https://doi.org/http://dx.doi.org/10.1016/j.tics.2010. 11.004.Crossref, Google Scholar
,2007. Response anticipation and response conflict: an event-related potential and functional magnetic resonance imaging study. J. Neurosci. 27, 2272–2282. https://doi.org/http://dx.doi.org/10.1523/ JNEUROSCI.3470-06.2007.Crossref, Google Scholar
,1998. Application of the directed transfer function method to mesial and lateral onset temporal lobe seizures. Brain Topogr. 11, 13–21.Crossref, Google Scholar
,2014. Who qualifies for deep brain stimulation for OCD? Data from a naturalistic clinical sample. J. Neuropsychiatr. Clin. Neurosci. 26, 81–86. https://doi.org/http://dx.doi.org/10.1176/appi.neuropsych.12090226.Crossref, Google Scholar
,2009. What did STAR*D teach us? Results from a large-scale, practical, clinical trial for patients with depression. Psychiatr. Serv. 60, 1439–1445. https://doi.org/http://dx.doi.org/10.1176/appi.ps.60.11.1439.Crossref, Google Scholar
,2005. A one-year comparison of vagus nerve stimulation with treatment as usual for treatment-resistant depression. Biol. Psychiatry 58, 364–373. https://doi.org/http://dx.doi.org/10.1016/j.biopsych.2005.07.028.Crossref, Google Scholar
,2010. Daily left prefrontal transcranial magnetic stimulation therapy for major depressive disorder: a sham-controlled randomized trial. Arch. Gen. Psychiatry 67, 507–516. https://doi.org/http://dx.doi.org/10.1001/archgenpsychiatry.2010.46.Crossref, Google Scholar
,2010a. Invasive circuitry-based neurotherapeutics: stereotactic ablation and deep brain stimulation for OCD. Neuropsychopharmocology 35, 317–336. https://doi.org/http://dx.doi.org/10.1038/npp.2009.128.Crossref, Google Scholar
,2010b. Deep brain stimulation of the ventral internal capsule/ventral striatum for obsessive-compulsive disorder: worldwide experience. Mol. Psychiatry 15, 64–79. https://doi.org/http://dx.doi.org/10.1038/mp.2008.55.Crossref, Google Scholar
,2013. Increased prefrontal cortex activity during negative emotion regulation as a predictor of depression symptom severity trajectory over 6 months. JAMA Psychiatry 70, 1181–1189. https://doi.org/http://dx.doi.org/10.1001/jamapsychiatry.2013.2430.Crossref, Google Scholar
,2012. A within-subject ERP and fMRI investigation of orientation-specific recognition memory for pictures. Cogn. Neurosci. 3, 174–192. https://doi.org/http://dx.doi.org/m1080/17588928.2012.669364.Crossref, Google Scholar
,2014 Sep. The prevalence of post-traumatic stress disorder (PTSD) in US combat soldiers: a head-to-head comparison of DSM-5 versus DSM-IV-TR symptom criteria with the PTSD checklist. Lancet Psychiatry 1 (4), 269–277.Crossref, Google Scholar
,2008. Assessing the economic costs of serious mental illness. Am. J. Psychiatry 165, 663–665. https://doi.org/http://dx.doi.org/10.1176/appi.ajp.2008.08030366.Crossref, Google Scholar
,2009. Disruptive insights in psychiatry: transforming a clinical discipline. J. Clin. Invest. 119, 700–705. https://doi.org/http://dx.doi.org/10.1172/JCI38832.Crossref, Google Scholar
,2010. Faulty circuits. Sci. Am. 302, 44–51. https://doi.org/http://dx.doi.org/10.1038/scientificamerican0410-44.Crossref, Google Scholar
,2010. Rethinking mental illness.J.Am. Med. Assoc. 303, 1970–1971. https://doi.org/http://dx.doi.org/10.1001/jama.2010.555.Crossref, Google Scholar
,2013. Prefrontal rTMS for treating depression: location and intensity results from the OPT-TMS multi-site clinical trial. Brain Stimul. 6, 108–117. https://doi.org/http://dx.doi.org/10. 1016/j.brs.2012.02.003.Crossref, Google Scholar
,2013. Comparative Effectiveness Review: Psychological Treatments and Pharmacological Treatments for Adults with Post-Traumatic Stress Disorder (PTSD).Google Scholar
,2010. Learning and cognitive flexibility: frontostriatal function and monoaminergic modulation. Curr. Opin. Neurobiol. 20, 199–204. https://doi.org/http://dx.doi.org/10.1016/j.conb.2010.01.007.Crossref, Google Scholar
,1973. Stereotactic limbic leucotomy: neurophysiological aspects and operative technique. Br. J. Psychiatry J. Ment. Sci. 123, 133–140.Crossref, Google Scholar
,2014. Thirty-day prevalence ofDSM-IV mental disorders among nondeployed soldiers in the US Army: results from the Army Study to Assess Risk and Resilience in Servicemembers (Army STARRS).JAMA Psychiatry 71, 504–513. https://doi.org/http://dx.doi.org/10.1001/jamapsychiatry.2014.28.Crossref, Google Scholar
,2010. Coalescence and fragmentation of cortical networks during focal seizures. J. Neurosci. 30, 10076–10085. https://doi.org/http://dx.doi.org/10.1523/JNEUROSCI.6309-09.2010.Crossref, Google Scholar
,2000. A quantitative study of gamma-band activity in human intracranial recordings triggered by visual stimuli. Eur. J. Neurosci. 12, 2608–2622. https://doi.org/http://dx.doi.org/10.1046/j.1460-9568.2000.00163.x.Crossref, Google Scholar
,2011. The NIMH-CATIE schizophrenia study: what did we learn? Am. J. Psychiatry 168, 770–775. https://doi.org/http://dx.doi.org/10.1176/appi.ajp.2011.11010039.Crossref, Google Scholar
,2005. Effectiveness of antipsychotic drugs in patients with chronic schizophrenia. N. Engl. J. Med. 353, 1209–1223. https://doi.org/http://dx.doi.org/10.1056/NEJMoa051688.Crossref, Google Scholar
,2013. Adaptive deep brain stimulation in advanced Parkinson disease. Ann. Neurol. 74, 449–457. https://doi.org/http://dx.doi.org/10.1002/ana.23951.Crossref, Google Scholar
,2009. Deep brain stimulation of the ventral capsule/ventral striatum for treatment-resistant depression. Biol. Psychiatry 65, 267–275. https://doi.org/http://dx.doi.org/10.1016Zj. biopsych.2008.08.029.Crossref, Google Scholar
,2014. Decoding spectrotemporal features of overt and covert speech from the human cortex. Front. Neuroengineering 7, 14. https://doi.org/http://dx.doi.org/10.3389/fneng.2014.00014.Crossref, Google Scholar
,2009. Targeted electrode-based modulation of neural circuits for depression.J. Clin. Invest.119, 717–725. https://doi.org/http://dx.doi.org/10.1172/JCI38454.Crossref, Google Scholar
,2014. In search of biomarkers in psychiatry: EEG-based measures of brain function. Am. J. Med. Genet. B Neuropsychiatr. Genet. 165, 111–121. https://doi.org/http://dx.doi.org/10.1002/ajmg.b.32208.Crossref, Google Scholar
,2009. Neurobiological basis of failure to recall extinction memory in posttraumatic stress disorder. Biol. Psychiatry 66, 1075–1082. https://doi.org/http://dx.doi.org/10.1016/j.biopsych.2009.06.026.Crossref, Google Scholar
,2001. An integrative theory of prefrontal cortex function. Annu. Rev. Neurosci. 24, 167–202.Crossref, Google Scholar
,2014. Deep brain stimulation for treatment-resistant depression: systematic review of clinical outcomes. Neurotherapeutics 11, 475–484. https://doi.org/http://dx.doi.org/10.1007/s13311-014-0282-1.Crossref, Google Scholar
,2011. Responsive cortical stimulation for the treatment of medically intractable partial epilepsy. Neurology 77, 1295–1304. https://doi.org/http://dx.doi.org/10.1212/WNL.0b013e3182302056.Crossref, Google Scholar
,2012. Dynamic causal modelling ofepileptic seizure propagation pathways: a combined EEG-fMRI study. NeuroImage 62, 1634–1642. https://doi.org/http://dx.doi.org/10.1016/j.neuroimage.2012.05.053.Crossref, Google Scholar
,2010. FieldTrip: open source software for advanced analysis of MEG, EEG, and invasive electrophysiological data. Comput. Intell. Neurosci. 2011, e156869. https://doi.org/http://dx.doi.org/10.1155/2011/156869.Google Scholar
,2010. A meta-analytic review of prolonged exposure for posttraumatic stress disorder. Clin. Psychol. Rev. 30, 635–641. https://doi.org/http://dx.doi.org/10.1016/j.cpr.2010.04.007.Crossref, Google Scholar
,2008. A mixed filter algorithm for cognitive state estimation from simultaneously recorded continuous and binary measures of performance. Biol. Cybern. 99, 1–14. https://doi.org/http://dx.doi.org/10. 1007/s00422-008-0227-z.Crossref, Google Scholar
,2001. Cerebral metabolic correlates as potential predictors of response to anterior cingulotomy for obsessive compulsive disorder. Biol. Psychiatry 50, 659–667.Crossref, Google Scholar
,2015. RIsk of suicide among us military service members following operation enduring freedom or operation Iraqi freedom deployment and separation from the US military.JAMA Psychiatry 72, 561–569. https://doi.org/http://dx.doi.org/10.1001/jamapsychiatry.2014.3195.Crossref, Google Scholar
,2013. DSM-5 field trials in the United States and Canada, part II: test-retest reliability of selected categorical diagnoses. Am.J. Psychiatry 170, 59–70. https://doi.org/http://dx.doi.org/10. 1176/appi.ajp.2012.12070999.Crossref, Google Scholar
,2013. Neural correlates of dysfunctional emotion regulation in major depressive disorder. A systematic review of neuroimaging studies. Neurosci. Biobehav. Rev. 37, 2529–2553. https://doi.org/http://dx.doi.org/10.1016/j.neubiorev.2013.07.018.Crossref, Google Scholar
,2011. Closed-loop deep brain stimulation is superior in ameliorating Parkinsonism. Neuron 72, 370–384. https://doi.org/http://dx.doi.org/10.1016/j.neuron.2011.08.023.Crossref, Google Scholar
,2011. Altered processing of contextual information during fear extinction in PTSD: an fMRI study. CNS Neurosci. Ther. 17, 227–236. https://doi.org/http://dx.doi.org/10.1111/j.1755-5949.2010.00152.x.Crossref, Google Scholar
,2015. Cortical information flow during flexible sensorimotor decisions. Science 348, 1352–1355. https://doi.org/http://dx.doi.org/10.1126/science.aab0551.Crossref, Google Scholar
,2003. Estimating a state-space model from point process observations. Neural Comput. 15, 965–991. https://doi.org/http://dx.doi.org/10.1162/089976603765202622.Crossref, Google Scholar
,2004. Dynamic analysis oflearning in behavioral experiments.J. Neurosci. 24, 447–461. https://doi.org/http://dx.doi.org/10.1523/JNEUROSCI.2908-03.2004.Crossref, Google Scholar
,2006. A state-space analysis for reconstruction of goal-directed movements using neural signals. Neural Comput. 18, 2465–2494. https://doi.org/http://dx.doi.org/10.1162/neco.2006.18.10.2465.Crossref, Google Scholar
,2014. Brain stimulation for epilepsy – local and remote modulation of network excitability. Brain Stimul. 7, 350–358. https://doi.org/http://dx.doi.org/10.1016/j.brs.2014.02.002.Crossref, Google Scholar
,2010. Prevalence of mental health problems and functional impairment among active component and National Guard soldiers 3 and 12 months following combat in Iraq. Arch. Gen. Psychiatry 67, 614–623. https://doi.org/http://dx.doi.org/10.1001/archgenpsychiatry.2010.54.Crossref, Google Scholar
,2015. Prolonged exposure vs eye movement desensitization and reprocessing vs waiting list for posttraumatic stress disorder in patients with a psychotic disorder: a randomized clinical trial. JAMA Psychiatry 72, 259. https://doi.org/http://dx.doi. org/10.1001/jamapsychiatry.2014.2637.Crossref, Google Scholar
,2002. Correspondence of event-related potential tomography and functional magnetic resonance imaging during language processing. Hum. Brain Mapp. 17, 4–12. https://doi.org/http://dx.doi.org/10.1002/hbm.10038.Crossref, Google Scholar
,2010. Evolving refractory major depressive disorder diagnostic and treatment paradigms: toward closed-loop therapeutics. Front. Neuroengineering 3, 7. https://doi.org/http://dx.doi.org/10.3389/fneng.2010.00007.Google Scholar
,2007. The STAR*D project results: a comprehensive review of findings. Curr. Psychiatry Rep. 9, 449–459. https://doi.org/http://dx.doi.org/10.1007/s11920-007-0061-3.Crossref, Google Scholar
,2015. An Implantable 64-Channel Neural Interface with Reconfigurable Recording and Stimulation. Eng. Med. Biol. Soc. EMBC. 37th Annu. Int. Conf. IEEE 2015. IEEE, pp. 7837–7840.Google Scholar
,2013. When optimism hurts: inflated predictions in psychiatric neuroimaging. Biol. Psychiatry https://doi.org/http://dx.doi.org/10.1016/j.biopsych.2013.05.014.Google Scholar
,2013. Global burden ofdisease attributable to mental and substance use disorders: findings from the global burden of disease study 2010. Lancet 382, 1575–1586. https://doi.org/http://dx.doi. org/10.1016/S0140-6736(13)61611-6.Crossref, Google Scholar
,2013. Baseline and treatment-emergent EEG biomarkers ofantidepressant medication response do not predict response to repetitive transcranial magnetic stimulation. Brain Stimul. 6, 929–931. https://doi.org/http://dx.doi.org/10. 1016/j.brs.2013.05.001.Crossref, Google Scholar
,2014. Affective brain-computer interfaces as enabling technology for responsive psychiatric stimulation. Brain-Comput Interfaces 1, 126–136. https://doi.org/http://dx.doi.org/m1080/2326263X.2014.912885.Crossref, Google Scholar
,2015. Deep brain stimulation for treatment-resistant psychiatric illnesses: what has gone wrong and what should we do next? Biol. Psychiatry https://doi.org/http://dx.doi.org/10.1016/j.biopsych.2015.06.005.Google Scholar
,2008. Estimation of time-varying connectivity patterns through the use of an adaptive directed transfer function. IEEE Trans. Biomed. Eng. 55, 2557–2564. https://doi.org/http://dx.doi.org/10.1109/TBME.2008.919885.Crossref, Google Scholar
,2013. Lesion analysis for cingulotomy and limbic leucotomy: comparison and correlation with clinical outcomes. J. Neurosurg. https://doi.org/http://dx.doi.org/10.3171/2013.9.JNS13839.Google Scholar
,2015. Cognitive State Prediction Using an EM Algorithm Applied to Gamma Distributed Data. Eng. Med. Biol. Soc. EMBC. 37th Annu. Int. Conf. IEEE 2015. IEEE, pp. 7819–7824.Crossref, Google Scholar
,